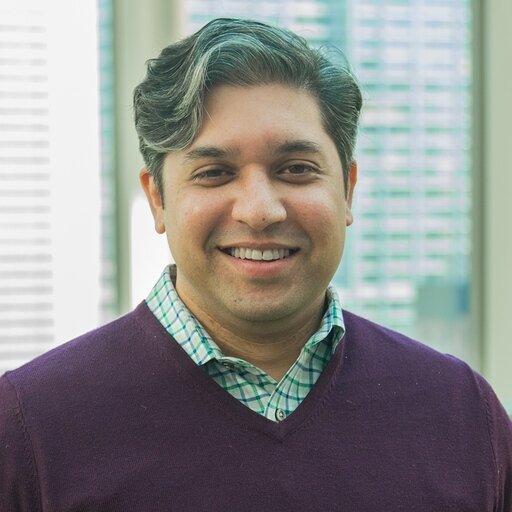
Sujoy Ganguly (Unity)
Talk: Customizable Computer Vision Expands Data Access Without Compromising Privacy
Abstract: In recent years, computer vision has made huge strides, helped by large-scale labeled datasets. However, these datasets had no guarantees or analysis diversity. Additionally, privacy concerns may limit the ability to collect more data. These problems are particularly acute in human-centric computer vision for AR/VR applications. An emerging alternative to real-world data that alleviates some of these issues is synthetic data. However, the creation of synthetic data generators is incredibly challenging and prevents researchers from exploring their usefulness. To promote research into the use of synthetic data, we release a set of data generators for computer vision. We found that pre-training a network using synthetic data and fine-tuning on real-world target data results in models that outperform models trained with the real data alone. Furthermore, we find remarkable gains when limited real-world data is available. Join us to learn how these freely available data generators should enable a wide range of research into the emerging field of simulation to real transfer learning for computer vision.
Bio: Sujoy Ganguly is the Head of Applied Machine Learning Research at Unity Technologies. Sujoy earned his Ph.D. in Applied Mathematics and Theoretical Physics from the University of Cambridge, understanding collective dynamics and transport phenomena in biological systems. After his Ph.D. Sujoy was a postdoctoral fellow at Yale University working in computational neuroscience. He has many years of industrial experience bringing the next generation of AI-driven technologies to market while publishing papers at major AI conferences. At Unity technologies, he is leading efforts in using simulated data to train AI that performs real-world tasks.
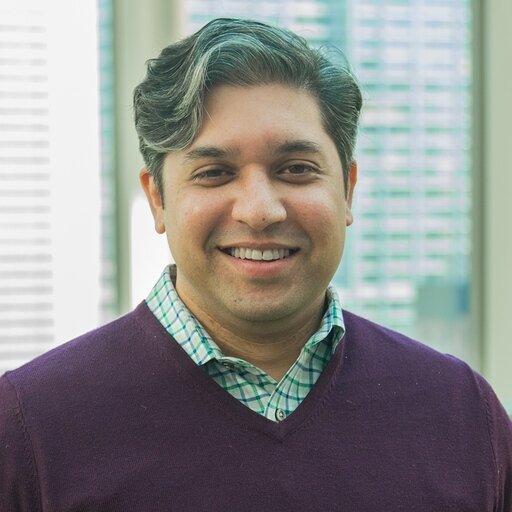